Multiprocessing with Pandas
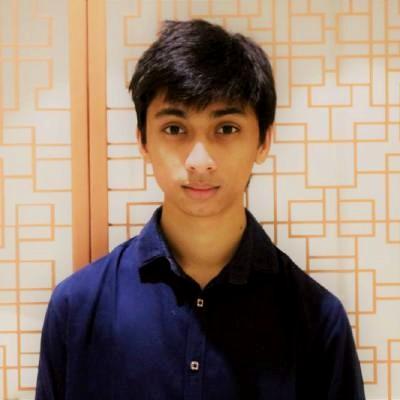
Multiprocessing is a powerful tool for improving the performance of data analysis tasks, and Pandas is a popular Python library for working with structured data. By leveraging the power of multiple CPU cores, multiprocessing allows Pandas to split data processing tasks across multiple processes, resulting in faster and more efficient computation. In this article, we will explore how to use multiprocessing with Pandas to speed up your data analysis workflow and improve the performance of your code.
In this post, we're going to use multiprocessing to process each subset of our dataframe parallelly.
A seperate core will process each group by seperately instead of doing it sequentially. This will increase the speed.
from multiprocessing import Pool
import pandas as pd
def take_mean_age(dataframe):
team, group = dataframe
return pd.DataFrame({
"Goals Scored": group["GF"].sum(),
"Goals Conceded": group["GA"].sum(),
"Total Points scored": group["Pts"].sum(),
"Final position of the team": group["Pos"].sum(),
"Win": group["W"].sum(),
"Draw": group["D"].sum(),
"Loss": group["L"].sum(),
}, index=[team])
pl = pd.read_csv('EPL Standings 2000-2022.csv', usecols=['Season', 'Pos', 'Team', 'Pld',
'W', 'D', 'L', 'GF', 'GA', 'GD', 'Pts'])
pl
Season | Pos | Team | Pld | W | D | L | GF | GA | GD | Pts | |
---|---|---|---|---|---|---|---|---|---|---|---|
0 | 2000-01 | 1 | Manchester United | 38 | 24 | 8 | 6 | 79 | 31 | 48 | 80 |
1 | 2000-01 | 2 | Arsenal | 38 | 20 | 10 | 8 | 63 | 38 | 25 | 70 |
2 | 2000-01 | 3 | Liverpool | 38 | 20 | 9 | 9 | 71 | 39 | 32 | 69 |
3 | 2000-01 | 4 | Leeds United | 38 | 20 | 8 | 10 | 64 | 43 | 21 | 68 |
4 | 2000-01 | 5 | Ipswich Town | 38 | 20 | 6 | 12 | 57 | 42 | 15 | 66 |
... | ... | ... | ... | ... | ... | ... | ... | ... | ... | ... | ... |
435 | 2021-22 | 16 | Everton | 38 | 11 | 6 | 21 | 43 | 66 | -23 | 39 |
436 | 2021-22 | 17 | Leeds United | 38 | 9 | 11 | 18 | 42 | 79 | -37 | 38 |
437 | 2021-22 | 18 | Burnley | 38 | 7 | 14 | 17 | 34 | 53 | -19 | 35 |
438 | 2021-22 | 19 | Watford | 38 | 6 | 5 | 27 | 34 | 77 | -43 | 23 |
439 | 2021-22 | 20 | Norwich City | 38 | 5 | 7 | 26 | 23 | 84 | -61 | 22 |
440 rows × 11 columns
with Pool(4) as p:
results = p.map(take_mean_age, pl.groupby("Team"))
results_df = pd.concat(results)
results_df.sort_values('Total Points scored', ascending=False)
Goals Scored | Goals Conceded | Total Points scored | Final position of the team | Win | Draw | Loss | |
---|---|---|---|---|---|---|---|
Manchester United | 1562 | 782 | 1698 | 63 | 507 | 177 | 152 |
Chelsea | 1538 | 757 | 1665 | 73 | 492 | 189 | 155 |
Arsenal | 1561 | 876 | 1603 | 83 | 470 | 193 | 173 |
Liverpool | 1516 | 808 | 1591 | 91 | 464 | 199 | 173 |
Manchester City | 1478 | 846 | 1440 | 126 | 428 | 156 | 214 |
Tottenham Hotspur | 1323 | 1011 | 1370 | 141 | 393 | 191 | 252 |
Everton | 1102 | 1059 | 1191 | 202 | 321 | 228 | 287 |
Newcastle United | 943 | 1100 | 973 | 222 | 260 | 193 | 307 |
West Ham United | 908 | 1060 | 895 | 225 | 238 | 181 | 303 |
Aston Villa | 866 | 1009 | 885 | 227 | 225 | 210 | 287 |
Southampton | 698 | 803 | 704 | 178 | 181 | 161 | 228 |
Fulham | 631 | 831 | 640 | 198 | 162 | 154 | 254 |
Leicester City | 583 | 592 | 554 | 114 | 150 | 104 | 164 |
Blackburn Rovers | 518 | 592 | 530 | 128 | 140 | 110 | 168 |
Sunderland | 520 | 795 | 520 | 215 | 127 | 139 | 266 |
Bolton Wanderers | 495 | 613 | 506 | 137 | 132 | 110 | 176 |
West Bromwich Albion | 510 | 772 | 490 | 197 | 117 | 139 | 238 |
Stoke City | 398 | 525 | 457 | 122 | 116 | 109 | 155 |
Crystal Palace | 428 | 545 | 437 | 131 | 115 | 92 | 173 |
Middlesbrough | 414 | 503 | 432 | 132 | 107 | 111 | 162 |
Wolverhampton Wanderers | 328 | 462 | 348 | 109 | 90 | 78 | 136 |
Wigan Athletic | 316 | 482 | 331 | 117 | 85 | 76 | 143 |
Charlton Athletic | 301 | 386 | 325 | 85 | 85 | 70 | 111 |
Burnley | 300 | 455 | 325 | 120 | 83 | 76 | 145 |
Swansea City | 306 | 383 | 312 | 85 | 82 | 66 | 118 |
Leeds United | 319 | 349 | 311 | 69 | 87 | 50 | 91 |
Birmingham City | 273 | 360 | 301 | 99 | 73 | 82 | 111 |
Portsmouth | 292 | 380 | 293 | 97 | 79 | 65 | 122 |
Watford | 275 | 441 | 261 | 113 | 67 | 60 | 139 |
Norwich City | 251 | 489 | 234 | 119 | 56 | 66 | 144 |
Bournemouth | 241 | 330 | 211 | 69 | 56 | 43 | 91 |
Brighton & Hove Albion | 190 | 258 | 209 | 72 | 48 | 65 | 77 |
Hull City | 181 | 323 | 171 | 88 | 41 | 48 | 101 |
Reading | 136 | 186 | 119 | 45 | 32 | 23 | 59 |
Sheffield United | 91 | 157 | 115 | 47 | 31 | 22 | 61 |
Ipswich Town | 98 | 106 | 102 | 23 | 29 | 15 | 32 |
Queens Park Rangers | 115 | 199 | 92 | 57 | 22 | 26 | 66 |
Derby County | 90 | 211 | 83 | 56 | 19 | 26 | 69 |
Cardiff City | 66 | 143 | 64 | 38 | 17 | 13 | 46 |
Huddersfield Town | 50 | 134 | 53 | 36 | 12 | 17 | 47 |
Brentford | 48 | 56 | 46 | 13 | 13 | 7 | 18 |
Blackpool | 55 | 78 | 39 | 19 | 10 | 9 | 19 |
Coventry City | 36 | 63 | 34 | 19 | 8 | 10 | 20 |
Bradford City | 30 | 70 | 26 | 20 | 5 | 11 | 22 |
Code snippet

Conclusion
Parallelize Pandas using Python's multiprocessing.